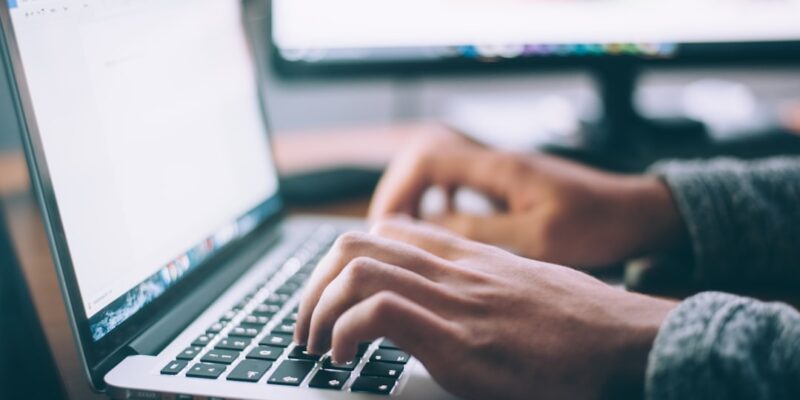
Revolutionizing Content Discovery with Algorithmic Recommendations
In today’s digital age, where there is an overwhelming amount of content available at our fingertips, the need for effective content discovery has become more important than ever. Algorithmic recommendations play a crucial role in helping users navigate through this vast sea of information and find content that is relevant and interesting to them.
Algorithmic recommendations can be defined as the use of mathematical algorithms and machine learning techniques to analyze user data and make personalized suggestions for content. These recommendations can be found in various platforms such as streaming services, e-commerce websites, social media platforms, and news aggregators. They aim to provide users with a tailored experience by suggesting content that aligns with their preferences and interests.
Key Takeaways
- Algorithmic recommendations use machine learning to suggest content to users.
- Personalized recommendations benefit both users and publishers by increasing engagement and revenue.
- Understanding the science behind algorithmic recommendations involves analyzing user data and behavior.
- Implementing algorithmic recommendations can be challenging due to data privacy concerns and technical limitations.
- Best practices for developing effective recommendation systems include testing and refining algorithms and considering user feedback.
The Role of Machine Learning in Revolutionizing Content Discovery
Machine learning is a subset of artificial intelligence that focuses on the development of algorithms and models that enable computers to learn and make predictions or decisions without being explicitly programmed. It has revolutionized content discovery by allowing algorithms to continuously learn from user behavior and adapt their recommendations accordingly.
Machine learning algorithms are used in algorithmic recommendations to analyze vast amounts of data, such as user preferences, browsing history, purchase history, and social interactions. These algorithms then use this data to identify patterns and make predictions about what content a user is likely to enjoy or find useful. The more data the algorithm has access to, the better it becomes at making accurate recommendations.
How Algorithmic Recommendations are Changing the Way We Consume Content
Algorithmic recommendations have completely transformed the way we consume content. They have made it easier for users to discover new content that they may not have otherwise come across. For example, streaming services like Netflix use algorithmic recommendations to suggest movies and TV shows based on a user’s viewing history and preferences. This allows users to explore a wider range of content and discover hidden gems that they may have missed.
Algorithmic recommendations also improve the user experience by reducing the time and effort required to find relevant content. Instead of manually searching for content, users can rely on the recommendations provided by the algorithm. This not only saves time but also ensures that users are presented with content that is tailored to their interests, increasing their overall satisfaction.
The Benefits of Personalized Recommendations for Users and Publishers
Benefits of Personalized Recommendations | Description |
---|---|
Increased User Engagement | Personalized recommendations keep users engaged by providing content that is relevant to their interests and preferences. |
Improved User Experience | Personalized recommendations enhance the user experience by making it easier for users to find content that they are interested in. |
Higher Click-Through Rates | Personalized recommendations have been shown to increase click-through rates, as users are more likely to click on content that is relevant to them. |
Increased Revenue for Publishers | Personalized recommendations can lead to increased revenue for publishers, as users are more likely to engage with and click on content that is relevant to them. |
Improved Content Discovery | Personalized recommendations help users discover new content that they may not have otherwise found, leading to a more diverse and engaging experience. |
Personalized recommendations offer numerous benefits for both users and publishers. For users, personalized recommendations improve engagement and retention. By suggesting content that aligns with a user’s preferences, algorithmic recommendations keep users coming back for more. This leads to increased user engagement and longer session times, which are key metrics for digital platforms.
For publishers, personalized recommendations can lead to increased revenue and customer loyalty. By suggesting relevant content to users, publishers can increase the chances of users making a purchase or consuming more content. This not only boosts revenue but also fosters a sense of loyalty among users, as they feel that the platform understands their preferences and caters to their needs.
Understanding the Science behind Algorithmic Recommendations
There are several recommendation algorithms used in algorithmic recommendations, with collaborative filtering being one of the most common. Collaborative filtering works by analyzing the behavior of similar users and making recommendations based on their shared interests. For example, if User A and User B have similar viewing histories on a streaming service, the algorithm may recommend a movie that User A has watched but User B hasn’t.
Other recommendation algorithms include content-based filtering, which analyzes the attributes of items to make recommendations, and hybrid filtering, which combines multiple algorithms to provide more accurate recommendations. These algorithms rely on data collected from users, such as ratings, reviews, and interactions, to make predictions about their preferences.
The Challenges of Implementing Algorithmic Recommendations in Content Discovery
While algorithmic recommendations offer numerous benefits, there are also challenges associated with their implementation. One of the main challenges is privacy concerns and data protection. To make accurate recommendations, algorithms need access to user data, which raises concerns about how this data is collected, stored, and used. It is important for platforms to be transparent about their data practices and ensure that user privacy is protected.
Another challenge is ensuring diversity and avoiding filter bubbles. Algorithmic recommendations have the potential to create echo chambers, where users are only exposed to content that aligns with their existing beliefs and interests. This can limit their exposure to new ideas and perspectives. To address this challenge, platforms need to incorporate diversity into their recommendation algorithms and provide users with options to explore content outside of their comfort zone.
Best Practices for Developing Effective Algorithmic Recommendation Systems
Developing effective algorithmic recommendation systems requires following best practices. Transparency and user control are key principles that should be prioritized. Users should have visibility into how their data is being used and the ability to control the recommendations they receive. This helps build trust and ensures that users feel in control of their own experience.
Regular testing and optimization are also crucial for developing effective recommendation systems. Algorithms should be continuously tested and refined based on user feedback and performance metrics. This allows platforms to improve the accuracy of their recommendations over time and ensure that users are receiving the most relevant content.
The Future of Content Discovery: Trends and Predictions
The future of content discovery is likely to be shaped by emerging technologies and trends. One such trend is the rise of voice assistants and smart speakers. As more users interact with digital platforms through voice commands, algorithmic recommendations will need to adapt to this new mode of interaction. Voice assistants can provide personalized recommendations based on a user’s voice profile, making content discovery even more seamless.
Integration with social media and messaging apps is another trend that is likely to shape the future of content discovery. Platforms can leverage social media data to make more accurate recommendations based on a user’s social connections and interests. Messaging apps can also be used to deliver personalized recommendations directly to users, making content discovery a more interactive and social experience.
Case Studies: Successful Examples of Algorithmic Recommendations in Action
Two successful examples of algorithmic recommendations in action are Netflix and Spotify. Netflix’s recommendation engine is known for its accuracy and ability to suggest content that users are likely to enjoy. By analyzing user viewing history, ratings, and interactions, Netflix’s algorithm provides personalized recommendations that keep users engaged and coming back for more.
Spotify’s Discover Weekly playlist is another example of effective algorithmic recommendations. Every week, Spotify creates a personalized playlist for each user based on their listening history and preferences. This playlist introduces users to new artists and songs that they may not have discovered otherwise, enhancing their music discovery experience.
The Importance of Algorithmic Recommendations in the Digital Age
In conclusion, algorithmic recommendations play a crucial role in content discovery in the digital age. They help users navigate through the vast amount of content available and find what is most relevant and interesting to them. Machine learning has revolutionized content discovery by enabling algorithms to continuously learn from user behavior and make personalized recommendations.
Algorithmic recommendations offer numerous benefits for both users and publishers. They improve engagement and retention for users, leading to increased revenue and customer loyalty for publishers. However, there are challenges associated with implementing algorithmic recommendations, such as privacy concerns and ensuring diversity. By following best practices and staying ahead of emerging trends, platforms can develop effective recommendation systems that enhance the content discovery experience for users.
FAQs
What are algorithmic content recommendations?
Algorithmic content recommendations are suggestions for content that are generated by algorithms based on a user’s past behavior, preferences, and other data points.
How do algorithmic content recommendations work?
Algorithmic content recommendations work by analyzing a user’s behavior, such as what they have clicked on or searched for in the past, and using that data to suggest other content that they may be interested in.
What are the benefits of algorithmic content recommendations?
Algorithmic content recommendations can help users discover new content that they may not have found otherwise, and can also help content providers increase engagement and retention by suggesting relevant content to users.
What are the potential drawbacks of algorithmic content recommendations?
One potential drawback of algorithmic content recommendations is that they can create filter bubbles, where users are only exposed to content that reinforces their existing beliefs and interests. Additionally, there are concerns about the ethical implications of using algorithms to make decisions about what content users see.
How are algorithmic content recommendations used in different industries?
Algorithmic content recommendations are used in a variety of industries, including e-commerce, social media, and news media. In e-commerce, they are used to suggest products that a user may be interested in purchasing. In social media, they are used to suggest posts or accounts that a user may want to follow. In news media, they are used to suggest articles that a user may want to read based on their interests.