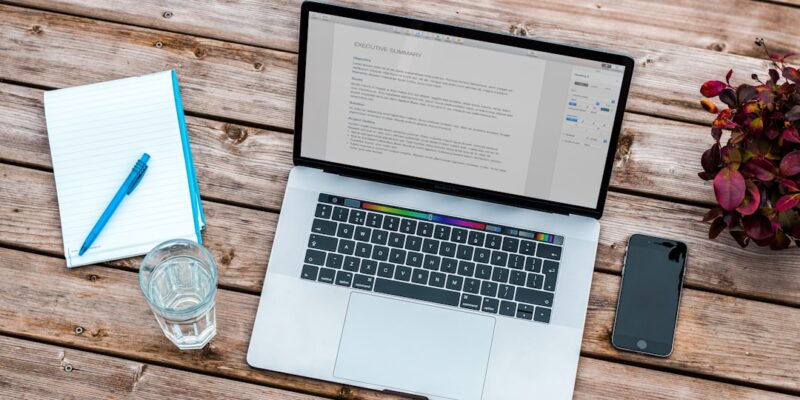
Revolutionizing E-Commerce: AI-Powered Personalized Recommendations
AI-powered personalized recommendations in e-commerce refer to the use of artificial intelligence technology to provide tailored product suggestions to individual customers based on their preferences, behavior, and past interactions with the website or app. These recommendations are designed to enhance the customer experience, increase engagement, and ultimately drive sales. In today’s highly competitive e-commerce landscape, personalized recommendations have become a crucial tool for businesses to stay ahead of the curve and meet the ever-increasing expectations of consumers.
Personalized recommendations are important in e-commerce because they offer a more personalized and relevant shopping experience for customers. By analyzing vast amounts of data, AI-powered recommendation engines can understand individual preferences and make accurate predictions about what products or services a customer is likely to be interested in. This not only saves customers time by presenting them with options that are most likely to appeal to them, but it also helps businesses increase customer satisfaction and loyalty.
Key Takeaways
- AI-powered personalized recommendations improve customer experience and increase sales in e-commerce.
- Data plays a crucial role in creating effective personalized recommendations.
- Understanding customer behavior is important for creating personalized recommendations.
- AI-powered recommendations can raise ethical concerns that need to be addressed.
- Best practices for implementing AI-powered personalized recommendations include testing and monitoring.
The Benefits of Personalized Recommendations for E-commerce Businesses
1. Increased customer engagement and loyalty: Personalized recommendations help businesses engage customers by providing them with relevant and interesting content. When customers feel that a website or app understands their preferences and needs, they are more likely to spend more time exploring the site, making repeat visits, and ultimately becoming loyal customers.
2. Higher conversion rates and sales: By presenting customers with products or services that align with their interests and preferences, personalized recommendations can significantly increase conversion rates. When customers see items that they are more likely to purchase, they are more likely to make a purchase, leading to increased sales for businesses.
3. Improved customer satisfaction and retention: Personalized recommendations can enhance the overall shopping experience for customers by making it easier for them to find what they are looking for. When customers feel that a website or app understands their needs and provides them with relevant suggestions, they are more likely to be satisfied with their experience and continue shopping with that business in the future.
How AI is Changing the Landscape of E-commerce
1. AI-powered recommendation engines: AI technology has revolutionized the way recommendations are generated in e-commerce. Recommendation engines use machine learning algorithms to analyze customer data and make predictions about what products or services a customer is likely to be interested in. These engines continuously learn and improve over time, providing increasingly accurate and personalized recommendations.
2. Machine learning algorithms: Machine learning algorithms are at the core of AI-powered recommendation engines. These algorithms analyze large amounts of data, including customer behavior, preferences, and past purchases, to identify patterns and make predictions about future behavior. By continuously learning from new data, these algorithms can adapt and improve their recommendations over time.
3. Natural language processing: Natural language processing (NLP) is another AI technology that is transforming e-commerce. NLP enables computers to understand and interpret human language, allowing businesses to provide personalized recommendations through chatbots and virtual assistants. These AI-powered assistants can understand customer queries and provide relevant product suggestions or answer questions in a conversational manner.
The Role of Data in Creating Effective Personalized Recommendations
Metrics | Description |
---|---|
Click-Through Rate (CTR) | The percentage of users who click on a recommended item after it is presented to them. |
Conversion Rate | The percentage of users who complete a desired action, such as making a purchase, after clicking on a recommended item. |
Average Order Value (AOV) | The average amount of money spent per order by users who click on recommended items. |
Customer Lifetime Value (CLV) | The total amount of money a customer is expected to spend over the course of their relationship with a business. |
Churn Rate | The percentage of customers who stop doing business with a company over a given period of time. |
1. Types of data used for recommendations: To create effective personalized recommendations, businesses need access to a wide range of data. This includes customer demographic information, browsing behavior, purchase history, product preferences, and feedback. By analyzing this data, businesses can gain insights into individual customer preferences and tailor their recommendations accordingly.
2. Importance of data quality and accuracy: The quality and accuracy of the data used for personalized recommendations are crucial for their effectiveness. Businesses need to ensure that the data they collect is accurate, up-to-date, and relevant to the recommendations being made. This requires implementing robust data collection processes, cleaning and validating the data, and regularly updating it to reflect changes in customer preferences.
3. Data privacy and security considerations: While data is essential for creating personalized recommendations, businesses must also prioritize data privacy and security. Customers need to trust that their personal information is being handled responsibly and securely. Businesses should implement strong data protection measures, obtain customer consent for data collection and use, and comply with relevant data privacy regulations.
The Importance of Understanding Customer Behavior for Personalized Recommendations
1. Customer segmentation: Customer segmentation involves dividing a customer base into distinct groups based on common characteristics or behaviors. By segmenting customers, businesses can better understand their preferences and needs, allowing them to provide more targeted and relevant recommendations. AI-powered recommendation engines can analyze customer data to identify meaningful segments and tailor recommendations accordingly.
2. Predictive analytics: Predictive analytics uses historical data and statistical algorithms to make predictions about future behavior. In the context of personalized recommendations, predictive analytics can be used to anticipate what products or services a customer is likely to be interested in based on their past behavior. By leveraging predictive analytics, businesses can provide recommendations that are more likely to resonate with individual customers.
3. Behavioral analysis: Behavioral analysis involves analyzing customer behavior, such as browsing patterns, click-through rates, and purchase history, to gain insights into their preferences and interests. By understanding how customers interact with a website or app, businesses can identify patterns and trends that can inform personalized recommendations. AI-powered recommendation engines can analyze vast amounts of behavioral data to make accurate predictions about customer preferences.
How AI-powered Recommendations Improve the Customer Experience
1. Personalized product recommendations: AI-powered recommendation engines can analyze customer data to provide personalized product recommendations based on individual preferences and behavior. These recommendations can be displayed on the website or app homepage, product pages, or through targeted email campaigns. By presenting customers with products that align with their interests, businesses can enhance the overall shopping experience and increase the likelihood of a purchase.
2. Dynamic pricing: AI technology can also be used to implement dynamic pricing strategies, where prices are adjusted in real-time based on factors such as demand, inventory levels, and customer behavior. By analyzing customer data, businesses can identify price sensitivity and offer personalized discounts or promotions to individual customers. This not only improves the customer experience but also increases the likelihood of a purchase.
3. Chatbots and virtual assistants: AI-powered chatbots and virtual assistants can provide personalized recommendations and assistance to customers in real-time. These AI-powered assistants can understand customer queries, provide relevant product suggestions, answer questions, and even assist with the purchasing process. By offering personalized and interactive support, businesses can enhance the customer experience and increase customer satisfaction.
The Impact of AI on Sales and Revenue in E-commerce
1. Case studies and examples: Numerous case studies have demonstrated the positive impact of AI-powered recommendations on sales and revenue in e-commerce. For example, Amazon attributes a significant portion of its revenue to its recommendation engine, which is estimated to generate 35% of its sales. Similarly, Netflix has reported that its personalized recommendation system is responsible for 80% of the content watched on its platform.
2. ROI of AI-powered recommendations: Implementing AI-powered personalized recommendations can yield a high return on investment (ROI) for e-commerce businesses. By increasing customer engagement, conversion rates, and sales, businesses can generate more revenue from their existing customer base. Additionally, personalized recommendations can also help businesses attract new customers by providing a unique and tailored shopping experience.
The Ethical Considerations of Using AI for Personalized Recommendations
1. Bias and discrimination: AI-powered recommendation systems are not immune to bias and discrimination. If the data used to train these systems is biased or reflects societal prejudices, the recommendations generated may perpetuate these biases. It is crucial for businesses to ensure that their recommendation engines are trained on diverse and representative data to avoid reinforcing discriminatory practices.
2. Transparency and accountability: AI-powered recommendation systems often operate as black boxes, making it difficult for users to understand how recommendations are generated. This lack of transparency can lead to mistrust and raise concerns about the fairness and accuracy of the recommendations. Businesses should strive to make their recommendation systems more transparent and accountable by providing explanations for the recommendations and allowing users to provide feedback.
3. Privacy and data protection: Personalized recommendations rely on the collection and analysis of large amounts of customer data. It is essential for businesses to prioritize data privacy and protection to maintain customer trust. This includes obtaining informed consent for data collection, implementing robust security measures, and complying with relevant data protection regulations.
The Future of AI-powered Personalized Recommendations in E-commerce
1. Advancements in AI technology: AI technology is continuously evolving, and advancements in areas such as deep learning, natural language processing, and computer vision are expected to further enhance the capabilities of personalized recommendation systems. These advancements will enable businesses to provide even more accurate, relevant, and personalized recommendations to customers.
2. Integration with other technologies: AI-powered personalized recommendations can be integrated with other emerging technologies to create even more immersive and personalized shopping experiences. For example, virtual reality (VR) and augmented reality (AR) can be used to allow customers to visualize products in their own environment before making a purchase. By combining AI with these technologies, businesses can create highly engaging and interactive shopping experiences.
3. Emerging trends and opportunities: As e-commerce continues to grow, there are several emerging trends and opportunities for AI-powered personalized recommendations. For example, voice-activated assistants such as Amazon’s Alexa or Apple’s Siri can be used to provide personalized recommendations through voice commands. Additionally, the rise of social commerce presents new opportunities for businesses to leverage AI-powered recommendations based on social media data.
Best Practices for Implementing AI-powered Personalized Recommendations in E-commerce
1. Choosing the right recommendation engine: There are various recommendation engine options available in the market, each with its own strengths and limitations. Businesses should carefully evaluate their needs and choose a recommendation engine that aligns with their goals and requirements. Factors to consider include scalability, ease of integration, customization options, and the ability to handle large amounts of data.
2. Collecting and analyzing data effectively: To create effective personalized recommendations, businesses need to collect and analyze data effectively. This involves implementing robust data collection processes, ensuring data quality and accuracy, and leveraging advanced analytics techniques to gain insights from the data. Businesses should also regularly update their data to reflect changes in customer preferences.
3. Testing and optimizing recommendations: Implementing AI-powered personalized recommendations is an iterative process that requires continuous testing and optimization. Businesses should regularly monitor the performance of their recommendation engine, analyze user feedback, and make adjustments as needed. A/B testing can be used to compare different recommendation strategies and identify the most effective approach.
4. Ensuring ethical and legal compliance: Businesses must ensure that their use of AI-powered personalized recommendations is ethical and compliant with relevant laws and regulations. This includes obtaining informed consent for data collection, implementing strong data protection measures, addressing bias and discrimination issues, and providing transparency and accountability in the recommendation process.
AI-powered personalized recommendations have become an essential tool for e-commerce businesses looking to enhance the customer experience, increase engagement, and drive sales. By leveraging AI technology, businesses can provide tailored product suggestions based on individual preferences and behavior, leading to higher conversion rates, increased customer satisfaction, and improved customer loyalty. However, it is crucial for businesses to consider the ethical implications of using AI for personalized recommendations, including issues of bias, transparency, privacy, and data protection. By implementing best practices and staying abreast of emerging trends in AI technology, e-commerce businesses can harness the power of personalized recommendations to stay ahead in a competitive market.
FAQs
What are AI-generated personalized product recommendations?
AI-generated personalized product recommendations are suggestions made by artificial intelligence algorithms based on a user’s past behavior, preferences, and other data points. These recommendations are tailored to each individual user and aim to provide a more personalized shopping experience.
How do AI-generated personalized product recommendations work?
AI-generated personalized product recommendations work by analyzing a user’s past behavior, such as their purchase history, browsing history, and search queries. The algorithm then uses this data to identify patterns and make predictions about what products the user is likely to be interested in. These recommendations are then presented to the user in various ways, such as on a website or in an email.
What are the benefits of AI-generated personalized product recommendations?
The benefits of AI-generated personalized product recommendations include increased customer engagement, higher conversion rates, and improved customer satisfaction. By providing users with relevant product suggestions, businesses can increase the likelihood of a purchase and build stronger relationships with their customers.
Are there any risks associated with AI-generated personalized product recommendations?
There are some risks associated with AI-generated personalized product recommendations, such as the potential for bias or privacy concerns. If the algorithm is not properly designed, it may inadvertently discriminate against certain groups of users. Additionally, there may be concerns about how user data is collected and used to generate these recommendations.
How can businesses implement AI-generated personalized product recommendations?
Businesses can implement AI-generated personalized product recommendations by using software or services that specialize in this area. These tools typically require access to user data, such as purchase history and browsing behavior, in order to generate accurate recommendations. Businesses may also need to work with data scientists or other experts to ensure that the algorithm is properly designed and implemented.