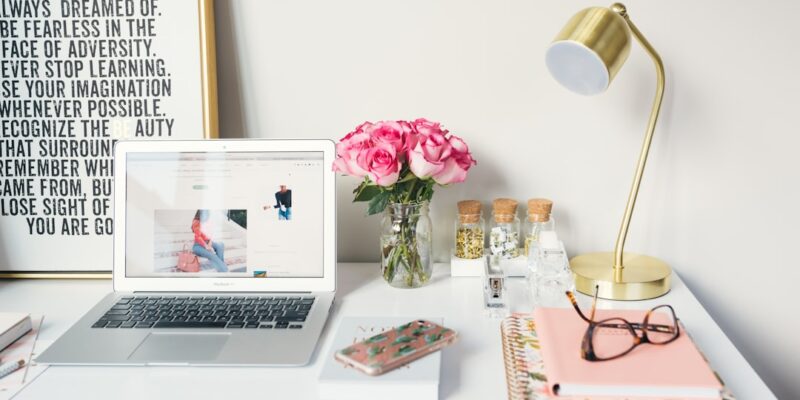
Revolutionizing E-Book Sales with Machine Learning
The e-book industry has experienced significant growth in recent years, revolutionizing the way people consume books. E-books offer convenience, accessibility, and a wide range of options for readers. With the rise of digital platforms and devices such as e-readers, tablets, and smartphones, e-books have become a popular choice for readers worldwide.
E-book sales play a crucial role in the publishing industry. They have become a significant source of revenue for publishers and authors alike. E-books offer higher profit margins compared to traditional print books, as they eliminate the costs associated with printing, distribution, and inventory management. This has made e-books an attractive option for publishers looking to maximize their profits.
Furthermore, e-book sales have opened up new opportunities for self-published authors. With the ease of publishing and distributing e-books through online platforms such as Amazon Kindle Direct Publishing and Smashwords, authors can reach a global audience without the need for a traditional publishing deal. This has democratized the publishing industry and allowed aspiring authors to showcase their work to a wider audience.
Key Takeaways
- Machine learning can help improve e-book sales by enhancing customer experience and personalizing recommendations.
- It can also be used to predict sales and enhance marketing strategies.
- However, implementing machine learning in e-book sales comes with its own set of challenges.
- The future of e-book sales is likely to be heavily influenced by machine learning technology.
- Overall, machine learning has the potential to revolutionize the e-book industry and improve sales for publishers and authors.
Understanding Machine Learning
Machine learning is a subset of artificial intelligence that focuses on developing algorithms and models that enable computers to learn from data and make predictions or decisions without being explicitly programmed. It involves training a computer system to recognize patterns in data and make informed decisions based on those patterns.
There are several types of machine learning techniques, including supervised learning, unsupervised learning, and reinforcement learning. In supervised learning, the computer is trained on labeled data, where it learns to predict or classify new data based on the patterns it has learned from the training data. Unsupervised learning involves training the computer on unlabeled data, where it learns to find patterns or group similar data points together. Reinforcement learning involves training the computer through trial and error, where it learns to make decisions based on feedback from its environment.
Machine learning has applications in various industries, including healthcare, finance, retail, and marketing. In healthcare, machine learning algorithms can be used to analyze medical images and detect diseases such as cancer. In finance, machine learning can be used to predict stock prices and make investment decisions. In retail, machine learning can be used to analyze customer data and make personalized product recommendations. In marketing, machine learning can be used to analyze customer behavior and optimize marketing campaigns.
The Role of Machine Learning in E-Book Sales
Machine learning plays a crucial role in e-book sales by enabling publishers and retailers to analyze customer data, improve marketing strategies, and personalize the reading experience for customers.
One way machine learning is used in e-book sales is through the analysis of customer data. By analyzing data such as purchase history, reading habits, and preferences, machine learning algorithms can identify patterns and trends that can help publishers and retailers understand their customers better. This information can then be used to tailor marketing campaigns, improve product recommendations, and optimize pricing strategies.
Several companies are already using machine learning in e-book sales. For example, Amazon uses machine learning algorithms to analyze customer data and make personalized product recommendations. Goodreads, a social cataloging website for book lovers, uses machine learning to analyze user ratings and reviews to provide personalized book recommendations. Kobo, an e-book retailer, uses machine learning algorithms to analyze customer data and optimize pricing strategies.
Benefits of Machine Learning in E-Book Sales
Benefits of Machine Learning in E-Book Sales |
---|
Increased sales |
Improved customer experience |
Personalized recommendations |
Efficient inventory management |
Reduced costs |
Improved marketing strategies |
Machine learning offers several benefits in e-book sales, including increased efficiency in sales and marketing, improved customer experience, and personalized recommendations.
One of the main benefits of machine learning in e-book sales is increased efficiency in sales and marketing. By analyzing customer data and identifying patterns and trends, machine learning algorithms can help publishers and retailers optimize their marketing campaigns. This includes targeting the right audience with the right message at the right time, optimizing pricing strategies based on demand and competition, and identifying potential customers who are likely to make a purchase.
Another benefit of machine learning in e-book sales is improved customer experience. By analyzing customer data and understanding their preferences, machine learning algorithms can provide personalized recommendations that are tailored to each individual customer. This not only enhances the reading experience for customers but also increases customer satisfaction and loyalty.
Personalized recommendations are another significant benefit of machine learning in e-book sales. By analyzing customer data and understanding their preferences, machine learning algorithms can recommend books that are likely to be of interest to each individual customer. This not only helps customers discover new books but also increases sales for publishers and retailers.
Improving Customer Experience with Machine Learning
Machine learning can enhance the customer experience in e-book sales by providing personalized recommendations, improving search functionality, and offering interactive features.
One way machine learning improves the customer experience is through personalized recommendations. By analyzing customer data and understanding their preferences, machine learning algorithms can recommend books that are likely to be of interest to each individual customer. This helps customers discover new books that they may not have found otherwise and increases their engagement with the platform.
Another way machine learning enhances the customer experience is by improving search functionality. Machine learning algorithms can analyze customer search queries and understand their intent, allowing for more accurate search results. This helps customers find the books they are looking for more quickly and easily, improving their overall experience with the platform.
Machine learning can also offer interactive features that enhance the reading experience for customers. For example, some e-book platforms use machine learning algorithms to analyze customer reading habits and provide personalized reading recommendations based on their interests. This helps customers discover new books and authors that align with their preferences, increasing their engagement with the platform.
Personalizing E-Book Recommendations with Machine Learning
Machine learning can personalize e-book recommendations by analyzing customer data, understanding their preferences, and recommending books that are likely to be of interest to each individual customer.
One way machine learning personalizes e-book recommendations is by analyzing customer data. By analyzing data such as purchase history, reading habits, and preferences, machine learning algorithms can identify patterns and trends that help understand each customer’s unique preferences. This information can then be used to recommend books that are likely to be of interest to each individual customer.
Another way machine learning personalizes e-book recommendations is by understanding customer preferences. Machine learning algorithms can analyze customer data and identify patterns and trends that help understand each customer’s unique preferences. This information can then be used to recommend books that align with each individual customer’s interests.
Several companies are already using machine learning for personalized e-book recommendations. For example, Amazon uses machine learning algorithms to analyze customer data and provide personalized product recommendations. Goodreads uses machine learning to analyze user ratings and reviews to provide personalized book recommendations. Kobo uses machine learning algorithms to analyze customer data and provide personalized book recommendations.
Enhancing E-Book Marketing Strategies with Machine Learning
Machine learning can improve e-book marketing strategies by analyzing customer data, optimizing marketing campaigns, and targeting the right audience with the right message at the right time.
One way machine learning enhances e-book marketing strategies is by analyzing customer data. By analyzing data such as purchase history, reading habits, and preferences, machine learning algorithms can identify patterns and trends that help understand each customer’s unique preferences. This information can then be used to tailor marketing campaigns and target the right audience with the right message at the right time.
Another way machine learning improves e-book marketing strategies is by optimizing marketing campaigns. Machine learning algorithms can analyze customer data and identify patterns and trends that help optimize marketing campaigns. This includes identifying the most effective marketing channels, optimizing pricing strategies based on demand and competition, and targeting potential customers who are likely to make a purchase.
Several companies are already using machine learning for e-book marketing. For example, Amazon uses machine learning algorithms to analyze customer data and optimize marketing campaigns. Goodreads uses machine learning to analyze user ratings and reviews to target potential customers with personalized book recommendations. Kobo uses machine learning algorithms to analyze customer data and optimize pricing strategies.
Predicting E-Book Sales with Machine Learning
Machine learning can predict e-book sales by analyzing historical sales data, identifying patterns and trends, and making informed predictions about future sales.
One way machine learning predicts e-book sales is by analyzing historical sales data. By analyzing data such as past sales, customer behavior, and market trends, machine learning algorithms can identify patterns and trends that help predict future sales. This information can then be used to make informed predictions about future sales and optimize inventory management.
Another way machine learning predicts e-book sales is by identifying patterns and trends. Machine learning algorithms can analyze historical sales data and identify patterns and trends that help predict future sales. This includes identifying seasonal trends, understanding customer behavior, and predicting the impact of marketing campaigns on sales.
Several companies are already using machine learning for sales predictions. For example, Amazon uses machine learning algorithms to analyze historical sales data and make informed predictions about future sales. Goodreads uses machine learning to analyze user ratings and reviews to predict the popularity of books. Kobo uses machine learning algorithms to analyze customer data and predict future sales.
Challenges in Implementing Machine Learning in E-Book Sales
While machine learning offers significant benefits in e-book sales, there are several challenges in implementing it effectively. These challenges include data quality and availability, algorithm selection, and privacy concerns.
One of the main challenges in implementing machine learning in e-book sales is data quality and availability. Machine learning algorithms require large amounts of high-quality data to train effectively. However, obtaining high-quality data can be challenging, as it requires accurate and up-to-date information about customers, books, and market trends. Additionally, accessing relevant data from different sources can be difficult, as data may be scattered across multiple platforms and systems.
Another challenge in implementing machine learning in e-book sales is algorithm selection. There are several machine learning algorithms available, each with its strengths and weaknesses. Selecting the right algorithm for a specific task can be challenging, as it requires a deep understanding of the problem domain and the available data. Additionally, fine-tuning and optimizing the selected algorithm can be time-consuming and resource-intensive.
Privacy concerns are also a significant challenge in implementing machine learning in e-book sales. Machine learning algorithms require access to customer data to make accurate predictions and recommendations. However, collecting and storing customer data raises privacy concerns, as it involves handling sensitive information such as purchase history, reading habits, and preferences. Ensuring the privacy and security of customer data is crucial to building trust with customers and complying with data protection regulations.
Future of E-Book Sales with Machine Learning
The future of e-book sales with machine learning looks promising, with potential advancements in technology that can further enhance the customer experience, improve marketing strategies, and predict sales accurately.
One potential advancement in machine learning technology for e-book sales is natural language processing (NLP). NLP involves training computers to understand and generate human language. With NLP, machine learning algorithms can analyze book content, customer reviews, and social media posts to gain insights into customer preferences and sentiment. This can help publishers and retailers understand customer preferences better and tailor their offerings accordingly.
Another potential advancement in machine learning technology for e-book sales is image recognition. Image recognition involves training computers to recognize and interpret images. With image recognition, machine learning algorithms can analyze book covers, author photos, and other visual elements to gain insights into customer preferences and trends. This can help publishers and retailers design more appealing book covers and marketing materials that resonate with customers.
Additionally, advancements in machine learning technology can lead to more accurate sales predictions. By analyzing larger datasets, incorporating more variables, and using more advanced algorithms, machine learning can make more accurate predictions about future sales. This can help publishers and retailers optimize inventory management, pricing strategies, and marketing campaigns, leading to increased sales and profitability.
In conclusion, machine learning plays a crucial role in e-book sales by enabling publishers and retailers to analyze customer data, improve marketing strategies, and personalize the reading experience for customers. Machine learning offers several benefits in e-book sales, including increased efficiency in sales and marketing, improved customer experience, and personalized recommendations. However, there are several challenges in implementing machine learning effectively, including data quality and availability, algorithm selection, and privacy concerns. Despite these challenges, the future of e-book sales with machine learning looks promising, with potential advancements in technology that can further enhance the customer experience, improve marketing strategies, and predict sales accurately.
FAQs
What is machine learning-powered e-book sales?
Machine learning-powered e-book sales is a system that uses artificial intelligence to analyze data and predict which e-books are likely to sell well. This system can help publishers and authors make informed decisions about which books to promote and how to market them.
How does machine learning-powered e-book sales work?
Machine learning-powered e-book sales works by analyzing data from various sources, such as sales history, customer reviews, and social media activity. The system uses this data to identify patterns and make predictions about which books are likely to sell well. Publishers and authors can then use this information to make informed decisions about which books to promote and how to market them.
What are the benefits of machine learning-powered e-book sales?
The benefits of machine learning-powered e-book sales include increased sales, improved marketing strategies, and better decision-making. By using artificial intelligence to analyze data, publishers and authors can identify which books are likely to sell well and focus their marketing efforts on those books. This can lead to increased sales and revenue.
Who can benefit from machine learning-powered e-book sales?
Publishers, authors, and e-book retailers can all benefit from machine learning-powered e-book sales. By using artificial intelligence to analyze data and make predictions about which books are likely to sell well, these stakeholders can make informed decisions about which books to promote and how to market them.
Is machine learning-powered e-book sales widely used?
Machine learning-powered e-book sales is becoming increasingly popular in the publishing industry. Many publishers and authors are using artificial intelligence to analyze data and make informed decisions about which books to promote and how to market them. However, it is not yet widely used by all publishers and authors.