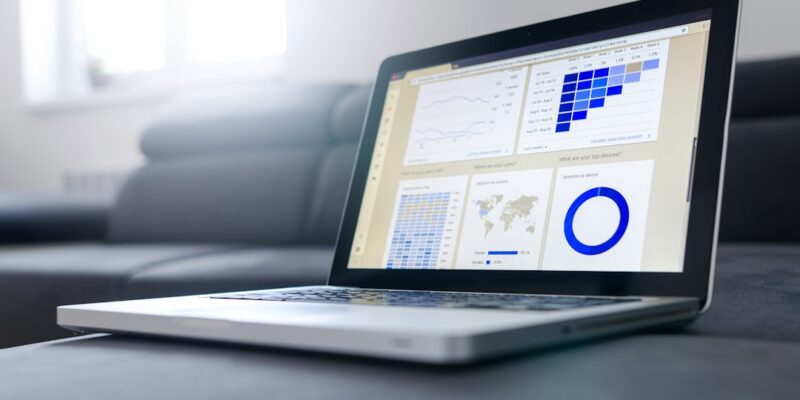
The Profitable Power of Data Science: Understanding the Economics Behind the Industry
Data science is a multidisciplinary field that combines statistical analysis, machine learning, and computer science to extract insights and knowledge from large volumes of data. In today’s digital age, where data is being generated at an unprecedented rate, data science has become increasingly important for businesses across industries. The economic impact of data science is significant, as it enables companies to make data-driven decisions, optimize operations, reduce costs, and drive revenue growth.
Data science plays a crucial role in the digital age by helping businesses make sense of the vast amounts of data they collect. With the proliferation of digital technologies and the internet, companies have access to an abundance of data from various sources such as social media, customer interactions, and sensor data. However, this data is often unstructured and complex, making it difficult to extract meaningful insights. Data science provides the tools and techniques to analyze and interpret this data, enabling businesses to gain valuable insights and make informed decisions.
The economic impact of data science is far-reaching. By leveraging data science techniques, companies can improve their operational efficiency, reduce costs, and drive revenue growth. Data-driven decision-making allows businesses to identify trends, patterns, and correlations in their data that can lead to more effective strategies and better outcomes. Additionally, data science enables companies to personalize their products and services based on customer preferences and behavior, leading to increased customer satisfaction and loyalty.
Key Takeaways
- Data science is an interdisciplinary field that combines statistics, computer science, and domain expertise to extract insights from data.
- Data science is crucial for businesses to stay competitive in today’s data-driven economy.
- Data scientists play a key role in driving profitability by identifying opportunities for revenue growth and cost reduction.
- The economic drivers of the data science industry include the increasing volume and variety of data, advances in technology, and the growing demand for data-driven insights.
- Understanding the value of data is essential for making informed business decisions and staying ahead of the competition.
The Importance of Data Science in Today’s Business Landscape
Data science is transforming industries across the board by enabling companies to gain a competitive edge through data-driven decision-making. Companies that embrace data science are able to leverage their data assets to gain insights into customer behavior, market trends, and operational efficiency. This allows them to make more informed decisions that drive profitability and growth.
One industry that has been greatly impacted by data science is retail. Companies like Amazon have used data science techniques to analyze customer browsing and purchasing behavior, allowing them to personalize product recommendations and optimize their supply chain. This has resulted in increased sales and customer satisfaction. Similarly, companies in the healthcare industry are using data science to analyze patient data and develop personalized treatment plans, leading to improved patient outcomes.
Data-driven decision-making also provides a competitive advantage for businesses. By analyzing data, companies can identify market trends and customer preferences, allowing them to develop targeted marketing campaigns and product offerings. This enables businesses to better understand their customers and deliver products and services that meet their needs. Companies that are able to effectively leverage data science have a distinct advantage over their competitors.
The Role of Data Scientists in Driving Profitability
Data scientists play a crucial role in driving profitability for businesses. They possess a unique set of skills and expertise that enable them to extract insights from complex data sets and translate them into actionable recommendations. Data scientists are proficient in statistical analysis, machine learning algorithms, and programming languages such as Python and R.
Data scientists contribute to business success by analyzing data to identify patterns, trends, and correlations that can inform decision-making. They use statistical techniques to uncover insights from large volumes of data, enabling businesses to make informed decisions based on evidence rather than intuition. By leveraging their expertise in machine learning algorithms, data scientists can develop predictive models that help businesses anticipate customer behavior, optimize operations, and mitigate risks.
The value of data-driven insights for profitability cannot be overstated. By using data science techniques, businesses can identify opportunities for cost reduction, revenue growth, and market expansion. For example, by analyzing customer data, companies can identify segments of customers who are more likely to churn and develop targeted retention strategies. Similarly, by analyzing sales data, companies can identify cross-selling and upselling opportunities that can drive revenue growth.
Key Economic Drivers of the Data Science Industry
Key Economic Drivers of the Data Science Industry | Description |
---|---|
Big Data | The massive amount of data generated by businesses and individuals that requires advanced analytics tools and techniques to extract insights and value. |
Artificial Intelligence | The development of intelligent machines that can perform tasks that typically require human intelligence, such as visual perception, speech recognition, decision-making, and language translation. |
Cloud Computing | The delivery of computing services, including servers, storage, databases, networking, software, analytics, and intelligence, over the internet. |
Internet of Things (IoT) | The network of physical devices, vehicles, home appliances, and other items embedded with electronics, software, sensors, and connectivity, which enables these objects to connect and exchange data. |
Cybersecurity | The protection of computer systems and networks from theft, damage, or unauthorized access, which is becoming increasingly important as more data is stored and transmitted online. |
Data Privacy | The protection of personal information and sensitive data from unauthorized access, use, or disclosure, which is a growing concern for individuals and businesses alike. |
Several key economic drivers have contributed to the growth of the data science industry. One of the main drivers is the exponential growth of big data. With the proliferation of digital technologies and the internet, companies have access to vast amounts of data from various sources. This data can provide valuable insights and competitive advantages if properly analyzed and interpreted. As a result, there is a growing demand for data scientists who can extract insights from big data and help businesses make data-driven decisions.
Another economic driver of the data science industry is the rise of machine learning and artificial intelligence (AI). Machine learning algorithms enable computers to learn from data and make predictions or take actions without being explicitly programmed. This has opened up new possibilities for businesses to automate processes, optimize operations, and develop personalized products and services. The increasing adoption of AI technologies across industries has created a demand for data scientists who can develop and deploy machine learning models.
The increasing demand for data-driven insights is also driving the growth of the data science industry. Businesses are realizing the value of data in informing decision-making and driving profitability. As a result, there is a growing need for data scientists who can analyze data, develop predictive models, and provide actionable insights. The demand for data scientists is expected to continue to grow as more companies recognize the importance of leveraging their data assets.
Understanding the Value of Data in Business Decision-Making
Data plays a crucial role in business decision-making by providing valuable insights that inform strategies and actions. However, it is important to recognize that not all data is created equal. The quality and accuracy of data are critical factors in ensuring that decisions are based on reliable information.
Data quality refers to the accuracy, completeness, consistency, and timeliness of data. High-quality data is essential for making accurate predictions and informed decisions. For example, if customer data is incomplete or inaccurate, it can lead to incorrect assumptions about customer behavior and preferences. Therefore, businesses need to invest in data quality management processes to ensure that their data is reliable and trustworthy.
Data also plays a crucial role in mitigating risk. By analyzing historical data and identifying patterns and trends, businesses can anticipate potential risks and take proactive measures to mitigate them. For example, by analyzing sales data, companies can identify seasonal fluctuations in demand and adjust their inventory levels accordingly. Similarly, by analyzing customer data, companies can identify potential fraud patterns and implement fraud detection measures.
The benefits of data-driven decision-making are numerous. By leveraging data, businesses can make more informed decisions that are based on evidence rather than intuition. This reduces the risk of making costly mistakes and increases the likelihood of success. Data-driven decision-making also enables businesses to identify opportunities for improvement and innovation, leading to increased efficiency and competitiveness.
The Impact of Data Science on Operational Efficiency and Cost Reduction
Data science has a significant impact on operational efficiency and cost reduction for businesses. By analyzing data, businesses can identify inefficiencies in their processes and develop strategies to optimize them. This can result in cost savings and improved productivity.
One way data science can optimize business processes is through predictive analytics. Predictive analytics uses historical data to develop models that can forecast future outcomes. By analyzing historical sales data, for example, businesses can develop predictive models that forecast future demand. This allows them to optimize their inventory levels and reduce carrying costs.
Data science can also help businesses reduce costs by identifying areas of waste or inefficiency. By analyzing operational data, businesses can identify bottlenecks or areas where resources are being underutilized. This allows them to make targeted improvements that increase efficiency and reduce costs.
Several companies have successfully used data science to reduce costs and improve operational efficiency. For example, UPS uses data science techniques to optimize its delivery routes, resulting in significant fuel savings. Similarly, Walmart uses data science to optimize its supply chain, reducing inventory carrying costs and improving product availability.
Leveraging Data Science to Drive Revenue Growth and Market Expansion
Data science is not only valuable for cost reduction and operational efficiency but also for driving revenue growth and market expansion. By analyzing customer data, businesses can identify new revenue streams and develop targeted marketing and sales strategies.
One way data science can drive revenue growth is through customer segmentation. By analyzing customer data, businesses can identify different segments of customers with distinct preferences and behaviors. This allows them to develop targeted marketing campaigns and product offerings that resonate with each segment. This can lead to increased customer acquisition, retention, and revenue.
Data science can also help businesses identify new market opportunities. By analyzing market data, businesses can identify emerging trends, customer needs, and gaps in the market. This allows them to develop new products or services that meet these needs and capture new market share.
Several companies have successfully used data science to drive revenue growth. For example, Netflix uses data science techniques to analyze customer viewing behavior and develop personalized recommendations. This has resulted in increased customer engagement and subscription revenue. Similarly, Uber uses data science to optimize its pricing algorithms, resulting in increased revenue per ride.
The Economics of Investing in Data Science: Costs and Benefits
Investing in data science initiatives can be costly, but the potential benefits outweigh the costs for businesses that are able to effectively leverage their data assets. The costs of implementing data science initiatives include hiring data scientists, investing in infrastructure and technology, and training employees.
Hiring data scientists can be expensive due to the high demand for their skills and expertise. Data scientists are in high demand across industries, and their salaries reflect this demand. Additionally, investing in infrastructure and technology can be costly, especially for businesses that need to upgrade their existing systems or invest in new technologies such as cloud computing or big data platforms.
Despite the costs, the potential return on investment for data science initiatives is significant. By leveraging data science techniques, businesses can make more informed decisions that drive profitability and growth. The insights gained from data analysis can lead to cost savings, revenue growth, and market expansion. Additionally, data-driven decision-making can lead to improved customer satisfaction and loyalty, resulting in long-term profitability.
Measuring the economic impact of data science can be challenging, but there are several metrics that businesses can use to assess the effectiveness of their data science initiatives. These metrics include cost savings, revenue growth, customer satisfaction, and market share. By tracking these metrics over time, businesses can evaluate the return on investment of their data science initiatives and make informed decisions about future investments.
The Future of Data Science: Trends and Opportunities for Profitability
The field of data science is constantly evolving, and there are several emerging trends that businesses should be aware of to leverage data science for profitability. One emerging trend is the increasing use of artificial intelligence (AI) and machine learning in data science. AI technologies enable computers to learn from data and make predictions or take actions without being explicitly programmed. This opens up new possibilities for businesses to automate processes, optimize operations, and develop personalized products and services.
Another emerging trend is the increasing use of big data analytics in data science. With the proliferation of digital technologies and the internet, companies have access to vast amounts of data from various sources. Big data analytics enables businesses to analyze and interpret this data to gain valuable insights. This can lead to improved decision-making, operational efficiency, and revenue growth.
Opportunities for businesses to leverage data science for profitability are abundant. One opportunity is in the healthcare industry, where data science can be used to analyze patient data and develop personalized treatment plans. Another opportunity is in the financial services industry, where data science can be used to detect fraud and identify investment opportunities. Additionally, businesses can leverage data science to optimize their supply chain, develop targeted marketing campaigns, and improve customer service.
The potential impact of future technological advancements on data science is significant. As technology continues to advance, businesses will have access to even more data and more powerful tools and techniques for analyzing and interpreting that data. This will enable businesses to gain even deeper insights and make even more informed decisions. Additionally, advancements in AI and machine learning will enable businesses to automate processes and develop intelligent systems that can learn and adapt.
The Profitable Power of Data Science in the Digital Age
In conclusion, data science plays a crucial role in the digital age by enabling businesses to make data-driven decisions, optimize operations, reduce costs, and drive revenue growth. The economic impact of data science is significant, as it provides businesses with valuable insights that inform strategies and actions. By leveraging data science techniques, businesses can gain a competitive edge by understanding customer behavior, market trends, and operational efficiency.
Investing in data science initiatives can be costly, but the potential benefits outweigh the costs for businesses that are able to effectively leverage their data assets. The insights gained from data analysis can lead to cost savings, revenue growth, and market expansion. Additionally, data-driven decision-making can lead to improved customer satisfaction and loyalty, resulting in long-term profitability.
The future of data science is promising, with emerging trends such as AI and big data analytics opening up new opportunities for businesses to leverage data science for profitability. As technology continues to advance, businesses will have access to even more data and more powerful tools and techniques for analyzing and interpreting that data. This will enable businesses to gain even deeper insights and make even more informed decisions.
In conclusion, businesses that invest in data science and embrace its power will be well-positioned for long-term success in the digital age. The profitable potential of data science is undeniable, and businesses that are able to effectively leverage their data assets will have a distinct advantage over their competitors.
If you’re interested in exploring the fascinating intersection of economics and data science, you won’t want to miss this insightful article by Wave Magnets. In their piece titled “The Economics of Data Science: Unlocking the Power of Information,” they delve into the profound impact that data science has on various industries and economies. From discussing the value of data as a strategic asset to examining the role of data scientists in driving innovation, this article offers valuable insights for anyone looking to understand the economic implications of data science. Check it out here and broaden your understanding of this dynamic field.
FAQs
What is data science?
Data science is an interdisciplinary field that involves the use of statistical and computational methods to extract insights and knowledge from data.
What is the economics of data science?
The economics of data science refers to the study of the economic principles and mechanisms that underlie the production, distribution, and consumption of data-driven products and services.
What are some examples of data-driven products and services?
Examples of data-driven products and services include search engines, social media platforms, e-commerce websites, online advertising, and personalized recommendations.
What are some of the economic challenges associated with data science?
Some of the economic challenges associated with data science include issues related to privacy, security, intellectual property, and market power.
How do data scientists create value?
Data scientists create value by using their skills and expertise to extract insights and knowledge from data that can be used to improve decision-making, optimize processes, and create new products and services.
What are some of the key skills required for a career in data science?
Some of the key skills required for a career in data science include programming, statistics, machine learning, data visualization, and communication.
What are some of the ethical considerations associated with data science?
Some of the ethical considerations associated with data science include issues related to privacy, bias, discrimination, and transparency. It is important for data scientists to be aware of these issues and to take steps to address them in their work.