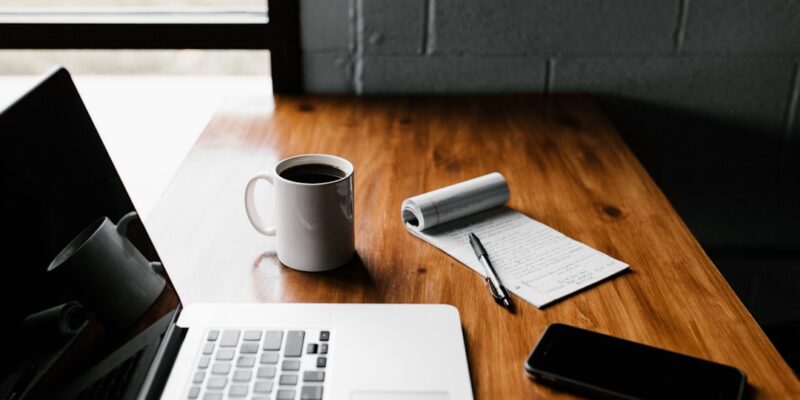
Revolutionizing E-Learning: Predictive Pricing for Online Courses
Predictive pricing is a revolutionary concept that is transforming the e-learning industry. It involves using data and algorithms to predict the optimal price for online courses, based on factors such as student demand, course content, and market conditions. Pricing plays a crucial role in e-learning, as it can impact student engagement, retention, and the overall success of online courses. In this blog post, we will explore the importance of pricing in e-learning and delve into the world of predictive pricing, discussing its advantages, implementation, and potential impact on the future of online learning.
Key Takeaways
- Predictive pricing is a new approach to pricing online courses that uses data analysis to determine the optimal price for each student.
- E-learning needs to be revolutionized to meet the changing needs of students and the demands of the modern workforce.
- Predictive pricing has the potential to be a game-changer for online learning, making it more accessible and affordable for students.
- Predictive pricing works by analyzing data on student behavior, preferences, and demographics to determine the optimal price for each course.
- The benefits of predictive pricing for online courses include increased revenue, higher student engagement, and improved student outcomes.
Understanding the Need for Revolutionizing E-Learning
Traditional e-learning models have faced several challenges in providing effective and personalized learning experiences to students. One of the main challenges is the one-size-fits-all approach, where courses are priced at a fixed rate regardless of individual student needs and preferences. This can lead to disengagement and low completion rates, as students may not find the course content relevant or valuable to their specific learning goals.
There is a growing need for personalized learning experiences that cater to the unique needs of each student. Predictive pricing has emerged as a solution to this problem by allowing course providers to offer dynamic pricing based on individual student profiles and preferences. By revolutionizing the way courses are priced, predictive pricing has the potential to transform e-learning into a more engaging and effective learning experience.
Predictive Pricing: A Game-Changer for Online Learning
Predictive pricing in e-learning refers to the use of data analytics and algorithms to determine the optimal price for online courses. It takes into account various factors such as student demand, course content, market conditions, and competitor pricing to predict the price that will maximize student engagement and retention while also ensuring profitability for course providers.
One of the key advantages of predictive pricing over traditional pricing models is its ability to offer personalized pricing based on individual student profiles. This allows course providers to offer discounts or incentives to students who may be more price-sensitive, while also maximizing revenue from students who are willing to pay a higher price for premium courses. By tailoring the price to each student’s needs and preferences, predictive pricing can significantly improve student engagement and retention rates.
How Predictive Pricing Works in E-Learning
Metrics | Description |
---|---|
Historical Data | The past performance of a course is analyzed to predict future demand and pricing. |
Market Trends | Current trends in the e-learning market are taken into consideration when setting prices. |
Competitor Analysis | Prices of similar courses offered by competitors are analyzed to ensure competitive pricing. |
Customer Segmentation | Prices are set based on the target audience and their willingness to pay. |
Dynamic Pricing | Prices are adjusted in real-time based on demand and availability. |
Predictive pricing in e-learning is based on a data-driven approach that involves analyzing large amounts of data to identify patterns and trends. Course providers collect data on student demographics, course preferences, learning outcomes, and other relevant factors to build predictive pricing algorithms. These algorithms use machine learning techniques to analyze the data and make predictions about the optimal price for each course.
Factors considered in predictive pricing include student demand, course popularity, competitor pricing, and market conditions. By analyzing these factors, predictive pricing algorithms can determine the price that will maximize student engagement and retention while also ensuring profitability for course providers.
There are several examples of predictive pricing algorithms used in e-learning. One example is the use of dynamic pricing, where the price of a course changes based on factors such as demand and availability. Another example is the use of personalized pricing, where each student is offered a unique price based on their individual profile and preferences. These algorithms are constantly evolving and improving as more data becomes available and new techniques are developed.
Benefits of Predictive Pricing for Online Courses
Predictive pricing offers several benefits for online courses, both for students and course providers. One of the main benefits is increased student engagement and retention. By offering personalized pricing based on individual student profiles, predictive pricing ensures that students find the course content relevant and valuable to their specific learning goals. This leads to higher engagement and completion rates, as students are more likely to stay motivated and committed to their studies.
Another benefit of predictive pricing is improved revenue for course providers. By optimizing the price based on factors such as student demand and market conditions, course providers can maximize their revenue while also ensuring that courses are accessible to a wide range of students. This allows course providers to invest in the development of high-quality courses and provide ongoing support and resources to students.
Predictive pricing also has the potential to enhance learning outcomes for students. By offering personalized pricing, course providers can attract a diverse range of students with different backgrounds, interests, and learning styles. This creates a more inclusive learning environment where students can learn from each other and benefit from a variety of perspectives and experiences.
Predictive Pricing vs Traditional Pricing Models: A Comparison
There are several differences between predictive pricing and traditional pricing models in e-learning. Traditional pricing models typically involve fixed prices for courses, regardless of individual student needs and preferences. This one-size-fits-all approach can lead to disengagement and low completion rates, as students may not find the course content relevant or valuable to their specific learning goals.
Predictive pricing, on the other hand, offers personalized pricing based on individual student profiles and preferences. This allows course providers to offer discounts or incentives to students who may be more price-sensitive, while also maximizing revenue from students who are willing to pay a higher price for premium courses. By tailoring the price to each student’s needs and preferences, predictive pricing can significantly improve student engagement and retention rates.
Both predictive pricing and traditional pricing models have their advantages and disadvantages. Traditional pricing models are simple and easy to implement, but they may not be effective in attracting and retaining students. Predictive pricing models, on the other hand, require more data and analysis, but they have the potential to offer a more personalized and engaging learning experience.
Case Studies: Successful Implementation of Predictive Pricing in E-Learning
There are several examples of e-learning platforms that have successfully implemented predictive pricing. One example is Coursera, a leading online learning platform that offers a wide range of courses from top universities and institutions. Coursera uses predictive pricing algorithms to determine the optimal price for each course, based on factors such as student demand, course popularity, and market conditions.
Through the use of predictive pricing, Coursera has been able to attract a diverse range of students and improve student engagement and retention rates. By offering personalized pricing based on individual student profiles, Coursera ensures that students find the course content relevant and valuable to their specific learning goals. This has led to higher completion rates and improved learning outcomes for students.
Another example is Udemy, an online learning platform that allows anyone to create and sell courses. Udemy uses predictive pricing algorithms to determine the optimal price for each course, based on factors such as course content, instructor reputation, and market conditions. By optimizing the price based on these factors, Udemy has been able to attract a wide range of students and maximize revenue for course creators.
Challenges and Limitations of Predictive Pricing in E-Learning
While predictive pricing offers many benefits for e-learning, there are also several challenges and limitations that need to be considered. One of the main challenges is the ethical considerations in using student data for pricing. Predictive pricing relies on collecting and analyzing large amounts of data on student demographics, preferences, and learning outcomes. There is a need to ensure that this data is collected and used in a responsible and ethical manner, with proper consent from students.
Another challenge is the accuracy of predictive pricing algorithms. While these algorithms are constantly evolving and improving, there is still a margin of error in their predictions. Course providers need to carefully monitor and evaluate the performance of these algorithms to ensure that they are providing accurate and reliable predictions.
There is also a potential for bias in predictive pricing algorithms. If the algorithms are not properly designed or trained, they may inadvertently discriminate against certain groups of students based on factors such as race, gender, or socioeconomic status. Course providers need to be aware of these potential biases and take steps to mitigate them, such as regularly auditing and testing the algorithms for fairness and accuracy.
Future of E-Learning with Predictive Pricing
The future of e-learning with predictive pricing looks promising. As technology continues to advance and more data becomes available, there is a potential for further innovation in e-learning pricing models. Predictive pricing algorithms can become more accurate and reliable, leading to better predictions and improved student engagement and retention rates.
There is also the potential for predictive pricing to be integrated with other emerging technologies, such as artificial intelligence and virtual reality. These technologies can enhance the learning experience by providing personalized recommendations, adaptive learning paths, and immersive simulations. By combining predictive pricing with these technologies, e-learning can become even more engaging and effective.
The impact of predictive pricing on the e-learning industry is expected to be significant. As more course providers embrace predictive pricing, there will be a shift towards more personalized and engaging learning experiences. This will lead to higher completion rates, improved learning outcomes, and a more inclusive and accessible e-learning environment.
Embracing Predictive Pricing for a Better E-Learning Experience
In conclusion, predictive pricing is revolutionizing the e-learning industry by offering personalized pricing based on individual student profiles and preferences. It has the potential to transform e-learning into a more engaging and effective learning experience, with higher student engagement and retention rates.
Course providers need to embrace predictive pricing and leverage the power of data analytics and algorithms to optimize their pricing strategies. By doing so, they can attract a diverse range of students, maximize revenue, and improve learning outcomes.
The future of e-learning with predictive pricing looks promising, with the potential for further innovation in pricing models and integration with emerging technologies. As technology continues to advance, we can expect to see even more personalized and immersive learning experiences that cater to the unique needs and preferences of each student.
FAQs
What is predictive pricing for online courses?
Predictive pricing for online courses is a pricing strategy that uses data analysis and machine learning algorithms to predict the optimal price for an online course based on various factors such as demand, competition, and customer behavior.
How does predictive pricing work?
Predictive pricing works by analyzing large amounts of data related to customer behavior, market trends, and competition to identify patterns and predict future outcomes. Machine learning algorithms are used to process this data and make predictions about the optimal price for an online course.
What are the benefits of predictive pricing for online courses?
The benefits of predictive pricing for online courses include increased revenue, improved customer satisfaction, and better market positioning. By using data analysis and machine learning algorithms to set prices, online course providers can optimize their pricing strategies and offer courses at the right price to attract and retain customers.
What factors are considered in predictive pricing for online courses?
Factors that are considered in predictive pricing for online courses include customer behavior, market trends, competition, course content, and pricing history. By analyzing these factors, machine learning algorithms can predict the optimal price for an online course that will maximize revenue and customer satisfaction.
How accurate is predictive pricing for online courses?
The accuracy of predictive pricing for online courses depends on the quality and quantity of data used to train the machine learning algorithms. Generally, the more data that is available, the more accurate the predictions will be. However, there are always uncertainties and variables that can affect the accuracy of predictive pricing models.