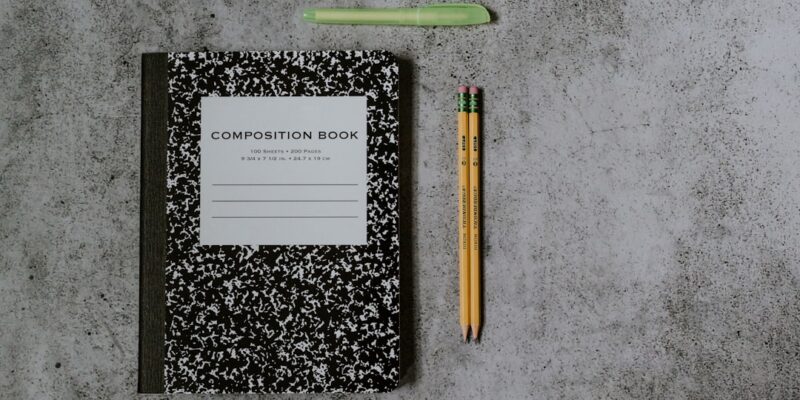
Revolutionize Your Sales with Machine Learning-Based Lead Scoring
Machine learning-based lead scoring is a powerful tool that can revolutionize the sales process. By using advanced algorithms and data analysis techniques, machine learning can accurately predict the likelihood of a lead converting into a customer. This allows sales teams to prioritize their efforts and focus on leads that are most likely to result in a sale. Lead scoring is crucial in sales because it helps sales teams identify the most promising leads and allocate their resources effectively.
Key Takeaways
- Machine learning-based lead scoring can help sales teams prioritize leads and increase efficiency.
- Benefits of machine learning in sales include improved accuracy, faster decision-making, and increased revenue.
- Machine learning can improve lead scoring accuracy by analyzing large amounts of data and identifying patterns.
- Data plays a crucial role in machine learning-based lead scoring, as it is used to train algorithms and make predictions.
- Implementing machine learning-based lead scoring requires careful planning and consideration of factors such as data quality and integration with existing systems.
The Benefits of Machine Learning in Sales
One of the key benefits of using machine learning in sales is increased efficiency and productivity. By automating the lead scoring process, sales teams can save time and effort that would otherwise be spent manually evaluating leads. This allows them to focus on more important tasks, such as building relationships with potential customers and closing deals. Machine learning also enables sales teams to handle a larger volume of leads, as the algorithms can quickly analyze and score leads at scale.
Another benefit of machine learning-based lead scoring is improved accuracy. Traditional lead scoring methods often rely on subjective criteria and human judgment, which can be prone to bias and error. Machine learning algorithms, on the other hand, are based on objective data and patterns, resulting in more accurate predictions. This allows sales teams to prioritize their efforts on leads that are most likely to convert, increasing their chances of success.
Machine learning also provides a better understanding of customer behavior and preferences. By analyzing large amounts of data, machine learning algorithms can identify patterns and trends that may not be immediately apparent to humans. This insight can help sales teams tailor their approach to individual leads, increasing the likelihood of a successful sale. For example, if the algorithm identifies that a certain type of lead is more likely to convert after receiving a personalized email, the sales team can adjust their strategy accordingly.
How Machine Learning Can Improve Lead Scoring Accuracy
Machine learning algorithms work by analyzing large amounts of data and identifying patterns and relationships. They use this information to make predictions and decisions. In the context of lead scoring, machine learning algorithms can analyze data such as demographic information, past purchase history, website behavior, and social media activity to determine the likelihood of a lead converting into a customer.
Compared to traditional lead scoring methods, machine learning-based lead scoring offers several advantages. Traditional methods often rely on manual evaluation and subjective criteria, which can be time-consuming and prone to bias. Machine learning algorithms, on the other hand, can process large amounts of data quickly and objectively, resulting in more accurate predictions.
There are many examples of how machine learning has improved lead scoring accuracy. For example, a study by Salesforce found that companies using machine learning-based lead scoring achieved a 30% increase in conversion rates compared to those using traditional methods. Another study by InsideSales.com found that machine learning algorithms were able to predict which leads were most likely to convert with 95% accuracy.
The Role of Data in Machine Learning-Based Lead Scoring
Metrics | Description |
---|---|
Accuracy | The percentage of correctly predicted leads |
Precision | The percentage of predicted positive leads that are actually positive |
Recall | The percentage of actual positive leads that are correctly predicted as positive |
F1 Score | The harmonic mean of precision and recall |
AUC-ROC | The area under the receiver operating characteristic curve, which measures the trade-off between true positive rate and false positive rate |
Confusion Matrix | A table that shows the number of true positives, true negatives, false positives, and false negatives |
Data is a crucial component of machine learning-based lead scoring. The quality and quantity of data used can greatly impact the accuracy and effectiveness of the algorithms. It is important to collect and analyze relevant data in order to make accurate predictions about leads.
There are several types of data that can be used in machine learning-based lead scoring. This includes demographic information, such as age, gender, and location, as well as behavioral data, such as website visits, email interactions, and social media activity. Other types of data that can be useful include past purchase history, customer feedback, and industry trends.
Collecting and analyzing data for lead scoring requires a systematic approach. It is important to gather data from multiple sources and ensure its accuracy and completeness. This may involve integrating different systems and platforms to gather data from various touchpoints. Once the data is collected, it needs to be cleaned and processed before it can be used for analysis. This may involve removing duplicates, correcting errors, and transforming the data into a format that can be used by the machine learning algorithms.
Implementing Machine Learning-Based Lead Scoring in Your Sales Process
Implementing machine learning-based lead scoring in your sales process requires careful planning and execution. Here are some steps to take when implementing this technology:
1. Define your goals: Before implementing machine learning-based lead scoring, it is important to clearly define your goals and objectives. What do you hope to achieve with this technology? How will it fit into your overall sales strategy? By clearly defining your goals, you can ensure that the implementation process is aligned with your business objectives.
2. Choose the right solution: There are many machine learning-based lead scoring solutions available on the market. It is important to choose a solution that is tailored to your specific needs and requirements. Consider factors such as ease of use, scalability, and integration capabilities when selecting a solution.
3. Integrate with existing sales processes: Machine learning-based lead scoring should be integrated with your existing sales processes and workflows. This may involve integrating the solution with your CRM system or other sales tools. By integrating the technology seamlessly into your existing processes, you can ensure a smooth transition and maximize its effectiveness.
4. Train and educate your sales teams: It is important to provide training and education to your sales teams when implementing machine learning-based lead scoring. This will help them understand how the technology works and how to use it effectively. Training should cover topics such as data analysis, interpreting lead scores, and adjusting sales strategies based on the predictions.
Real-Life Examples of Machine Learning-Based Lead Scoring Success
There are many real-life examples of companies that have successfully implemented machine learning-based lead scoring and achieved significant results. One such example is HubSpot, a leading marketing and sales software company. HubSpot used machine learning algorithms to analyze data from millions of leads and identify patterns that indicated a high likelihood of conversion. This allowed their sales teams to focus on the most promising leads and increase their conversion rates.
Another example is IBM, which used machine learning-based lead scoring to improve the efficiency and effectiveness of their sales process. By analyzing data from various sources, including social media, website behavior, and past purchase history, IBM was able to identify the most promising leads and allocate their resources accordingly. This resulted in a significant increase in sales productivity and revenue.
Overcoming Challenges in Adopting Machine Learning-Based Lead Scoring
While machine learning-based lead scoring offers many benefits, there are also challenges and obstacles that organizations may face when adopting this technology. Some common challenges include:
1. Data quality: Machine learning algorithms rely on high-quality data to make accurate predictions. If the data used for lead scoring is incomplete or inaccurate, it can lead to inaccurate predictions and poor results. It is important to ensure that the data used for lead scoring is clean, complete, and up-to-date.
2. Resistance to change: Implementing machine learning-based lead scoring may require changes to existing processes and workflows. This can sometimes be met with resistance from sales teams who are comfortable with the status quo. It is important to address any concerns or resistance early on and provide training and support to help sales teams adapt to the new technology.
3. Integration with existing systems: Integrating machine learning-based lead scoring with existing systems and platforms can be a complex process. It may require technical expertise and coordination between different teams or departments. It is important to plan and execute the integration carefully to ensure a smooth transition.
To overcome these challenges, organizations can take several strategies. This includes investing in data quality management tools and processes, providing training and support to sales teams, and working closely with IT departments to ensure seamless integration.
The Future of Sales with Machine Learning-Based Lead Scoring
The future of sales with machine learning-based lead scoring is promising. As technology continues to advance, we can expect to see even more sophisticated algorithms and techniques that can further improve lead scoring accuracy and effectiveness. Some potential advancements and innovations include:
1. Predictive analytics: Machine learning algorithms can already make predictions about the likelihood of a lead converting into a customer. In the future, we can expect to see even more advanced predictive analytics capabilities, such as predicting the lifetime value of a customer or the likelihood of churn.
2. Personalization: Machine learning algorithms can analyze large amounts of data to identify patterns and trends. In the future, we can expect to see even more personalized sales experiences, where sales teams can tailor their approach to individual leads based on their preferences and behavior.
3. Automation: Machine learning-based lead scoring already automates the process of evaluating leads and predicting their likelihood of conversion. In the future, we can expect to see even more automation in the sales process, such as automated follow-ups and personalized recommendations.
Choosing the Right Machine Learning-Based Lead Scoring Solution for Your Business
When choosing a machine learning-based lead scoring solution for your business, there are several factors to consider. These include:
1. Ease of use: The solution should be easy to use and understand, even for non-technical users. It should have a user-friendly interface and provide clear instructions and guidance.
2. Scalability: The solution should be able to handle large volumes of data and scale as your business grows. It should be able to process and analyze data quickly and efficiently.
3. Integration capabilities: The solution should be able to integrate with your existing systems and platforms, such as your CRM system or marketing automation software. This will ensure a seamless transition and maximize the effectiveness of the technology.
4. Customization options: The solution should allow you to customize the lead scoring criteria and algorithms to fit your specific needs and requirements. This will ensure that the predictions are tailored to your business and industry.
When comparing different solutions on the market, it is important to evaluate them based on these factors and choose the one that best meets your needs.
Best Practices for Maximizing the Benefits of Machine Learning-Based Lead Scoring
To maximize the benefits of machine learning-based lead scoring, organizations can follow these best practices:
1. Continuously improve and optimize: Machine learning algorithms learn from data and improve over time. It is important to continuously analyze and evaluate the performance of the algorithms and make adjustments as needed. This may involve refining the lead scoring criteria, collecting additional data, or adjusting sales strategies based on the predictions.
2. Collaborate between sales and marketing teams: Machine learning-based lead scoring requires collaboration between sales and marketing teams. It is important to align goals and objectives, share data and insights, and work together to optimize the lead scoring process.
3. Monitor and measure results: It is important to monitor and measure the results of machine learning-based lead scoring to ensure its effectiveness. This may involve tracking conversion rates, revenue generated, or other key performance indicators. By regularly reviewing the results, organizations can identify areas for improvement and make data-driven decisions.
In conclusion, machine learning-based lead scoring is a powerful tool that can revolutionize the sales process. By using advanced algorithms and data analysis techniques, organizations can accurately predict the likelihood of a lead converting into a customer. This allows sales teams to prioritize their efforts and focus on leads that are most likely to result in a sale. By implementing machine learning-based lead scoring, organizations can increase efficiency and productivity, improve accuracy in lead scoring, and gain a better understanding of customer behavior and preferences. With careful planning and execution, organizations can overcome challenges and maximize the benefits of this technology in their sales process.
FAQs
What is machine learning-based lead scoring?
Machine learning-based lead scoring is a process of using machine learning algorithms to analyze and score leads based on their behavior, demographics, and other relevant data.
How does machine learning-based lead scoring work?
Machine learning-based lead scoring works by analyzing large amounts of data to identify patterns and trends that can be used to predict which leads are most likely to convert. The algorithms used in this process are trained on historical data to learn which factors are most important in predicting conversion.
What are the benefits of machine learning-based lead scoring?
The benefits of machine learning-based lead scoring include increased efficiency and accuracy in identifying high-quality leads, improved sales productivity, and better alignment between sales and marketing teams.
What types of data are used in machine learning-based lead scoring?
Machine learning-based lead scoring can use a variety of data sources, including demographic data, behavioral data, firmographic data, and engagement data.
What are some common machine learning algorithms used in lead scoring?
Some common machine learning algorithms used in lead scoring include logistic regression, decision trees, random forests, and neural networks.
How can machine learning-based lead scoring be implemented?
Machine learning-based lead scoring can be implemented using a variety of tools and platforms, including marketing automation software, customer relationship management (CRM) systems, and specialized lead scoring software. Implementation typically involves integrating data sources, training the machine learning algorithms, and setting up workflows for lead management and follow-up.
I don’t think the title of your article matches the content lol. Just kidding, mainly because I had some doubts after reading the article.