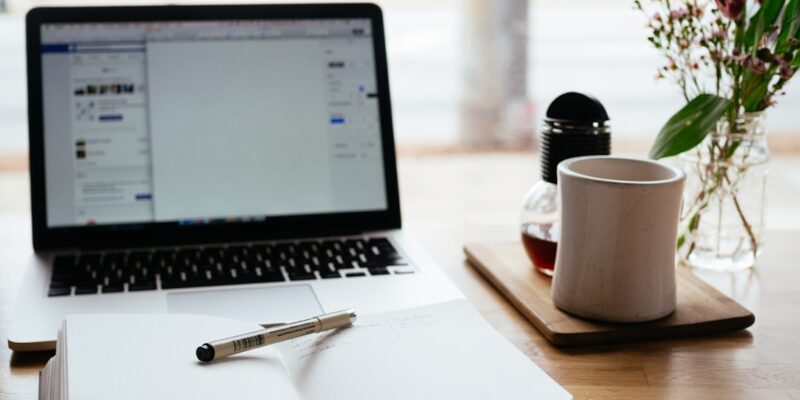
Revolutionizing Influencer Marketing with Machine Learning
Influencer marketing has become a popular strategy for brands to reach their target audience and promote their products or services. It involves partnering with individuals who have a large following on social media platforms, such as Instagram or YouTube, to create content that promotes the brand. These influencers have the ability to sway the opinions and purchasing decisions of their followers, making them valuable assets for brands.
Machine learning, on the other hand, is a subset of artificial intelligence that focuses on the development of algorithms and models that can learn from and make predictions or decisions based on data. It involves training these algorithms on large datasets to recognize patterns and make accurate predictions or decisions without being explicitly programmed.
Key Takeaways
- Influencer marketing and machine learning are two powerful tools that can be used together to enhance marketing strategies.
- Machine learning can help identify the right influencers for a campaign by analyzing data and predicting outcomes.
- Data analytics is crucial in influencer marketing as it helps measure the success of a campaign and identify areas for improvement.
- Using machine learning in influencer marketing can lead to more efficient and effective campaigns, saving time and resources.
- Predictive analytics can help forecast the success of a campaign and adjust strategies accordingly.
The Role of Machine Learning in Influencer Marketing
Machine learning can play a crucial role in influencer marketing by helping brands identify and select the most suitable influencers for their campaigns. With the vast amount of data available on social media platforms, it can be challenging for brands to manually analyze and identify influencers who align with their brand values and target audience. Machine learning algorithms can automate this process by analyzing data such as engagement rates, follower demographics, and content relevance to identify influencers who are likely to have a positive impact on a brand’s campaign.
There are several machine learning algorithms that can be used in influencer marketing. One example is the collaborative filtering algorithm, which analyzes user behavior and preferences to recommend influencers who are similar to those that a user already follows or engages with. Another example is the decision tree algorithm, which uses a series of if-then statements to classify influencers based on specific criteria such as follower count or engagement rate.
Understanding Data Analytics in Influencer Marketing
Data analytics plays a crucial role in influencer marketing as it provides valuable insights into the performance of campaigns and helps brands make informed decisions. It involves collecting and analyzing data from various sources such as social media platforms, website analytics, and customer feedback.
There are different types of data used in influencer marketing. One type is demographic data, which includes information about the age, gender, location, and interests of an influencer’s followers. This data can help brands determine whether an influencer’s audience aligns with their target audience. Another type is engagement data, which includes metrics such as likes, comments, and shares. This data can help brands assess the level of engagement an influencer’s content generates and determine their effectiveness in driving brand awareness or conversions.
Benefits of Using Machine Learning in Influencer Marketing
Benefits of Using Machine Learning in Influencer Marketing |
---|
Improved Targeting |
Increased Engagement |
Enhanced ROI |
Efficient Campaign Management |
Real-time Performance Tracking |
Optimized Content Creation |
Reduced Fraudulent Activities |
Using machine learning in influencer marketing offers several benefits for brands. Firstly, it increases efficiency and accuracy in influencer selection. Machine learning algorithms can analyze large amounts of data quickly and accurately, saving brands time and resources in manually identifying influencers. This also reduces the risk of human error and bias in the selection process.
Secondly, machine learning improves campaign performance and return on investment (ROI). By analyzing data from previous campaigns and identifying patterns, machine learning algorithms can make predictions about the success of future campaigns. This allows brands to optimize their strategies and allocate resources more effectively to achieve better results.
Lastly, machine learning enhances personalization and customization in influencer marketing. By analyzing data about an influencer’s audience and preferences, machine learning algorithms can help brands create personalized campaigns that resonate with their target audience. This leads to higher engagement rates and better brand-consumer relationships.
Predictive Analytics: Forecasting Influencer Marketing Outcomes
Predictive analytics is a branch of data analytics that uses historical data to make predictions about future outcomes. In influencer marketing, machine learning can be used for predictive analytics to forecast the outcomes of campaigns.
By analyzing historical data from previous campaigns, machine learning algorithms can identify patterns and trends that can help predict the success of future campaigns. For example, if a brand has run multiple campaigns with different influencers and has data on the engagement rates and conversions generated by each campaign, a machine learning algorithm can analyze this data to identify factors that contribute to campaign success. It can then use this information to predict the success of future campaigns based on the influencers selected and the content created.
Using predictive analytics in influencer marketing offers several benefits for brands. Firstly, it allows them to make more informed decisions about which influencers to partner with and what type of content to create. This reduces the risk of investing in campaigns that may not generate the desired results.
Secondly, predictive analytics helps brands allocate their resources more effectively. By predicting the outcomes of different campaigns, brands can prioritize their investments and focus on strategies that are likely to yield the highest returns.
Machine Learning Algorithms for Influencer Identification
There are several machine learning algorithms that can be used for influencer identification. One popular algorithm is the k-nearest neighbors (KNN) algorithm, which classifies influencers based on their similarity to other influencers in terms of follower demographics, engagement rates, or content relevance. The algorithm calculates the distance between an influencer and its neighbors and assigns it to the class with the majority of its neighbors.
Another algorithm is the support vector machine (SVM) algorithm, which creates a hyperplane that separates influencers into different classes based on specific criteria such as follower count or engagement rate. The algorithm aims to maximize the margin between the hyperplane and the influencers, making it an effective algorithm for binary classification tasks.
A third algorithm is the random forest algorithm, which combines multiple decision trees to make predictions about influencer suitability. Each decision tree in the random forest is trained on a subset of the data and makes a prediction based on its own set of rules. The final prediction is then determined by aggregating the predictions of all the decision trees.
Each machine learning algorithm has its own pros and cons. The KNN algorithm is simple and easy to implement, but it can be computationally expensive and sensitive to the choice of k, the number of neighbors to consider. The SVM algorithm is effective for binary classification tasks, but it can be sensitive to the choice of hyperparameters and may not perform well with imbalanced datasets. The random forest algorithm is robust and can handle large datasets, but it can be difficult to interpret and may overfit the data if not properly tuned.
Enhancing Campaign Performance with Machine Learning
Machine learning can be used to enhance campaign performance in influencer marketing by optimizing various aspects of a campaign. For example, machine learning algorithms can analyze data from previous campaigns to identify the most effective influencers, content types, or posting times. This information can then be used to optimize future campaigns and increase their chances of success.
One way machine learning can optimize campaign performance is by identifying influencers who are likely to generate high engagement rates or conversions. By analyzing historical data on influencers’ performance metrics, machine learning algorithms can identify patterns and characteristics that contribute to campaign success. This allows brands to select influencers who are more likely to generate positive results and maximize their return on investment.
Another way machine learning can optimize campaign performance is by identifying the most effective content types or formats. By analyzing data on engagement rates or conversions for different types of content, machine learning algorithms can identify patterns and trends that indicate which content types are more likely to resonate with an influencer’s audience. This allows brands to create content that is more likely to generate high engagement rates and drive conversions.
Personalization and Customization in Influencer Marketing with Machine Learning
Machine learning can be used to personalize influencer marketing campaigns by analyzing data about an influencer’s audience and preferences. By understanding the demographics, interests, and behaviors of an influencer’s followers, machine learning algorithms can help brands create content that is tailored to their target audience.
For example, if an influencer has a predominantly female audience between the ages of 18-24, machine learning algorithms can analyze data on the preferences and interests of this demographic to identify content themes or topics that are likely to resonate with them. This allows brands to create content that is more relevant and engaging, increasing the chances of driving conversions or brand loyalty.
Personalized campaigns have several benefits for brands. Firstly, they increase the chances of capturing the attention and interest of the target audience. By creating content that is tailored to their preferences and interests, brands can create a stronger connection with their audience and increase the chances of driving engagement or conversions.
Secondly, personalized campaigns can help brands stand out in a crowded marketplace. With the rise of influencer marketing, consumers are constantly bombarded with branded content. By creating personalized campaigns that speak directly to their target audience, brands can differentiate themselves from competitors and increase their chances of capturing attention and driving brand awareness.
Automating Influencer Marketing with Machine Learning
Machine learning can be used to automate various tasks in influencer marketing, saving brands time and resources. For example, machine learning algorithms can automate the process of influencer identification by analyzing data from social media platforms to identify influencers who align with a brand’s target audience and values.
By automating this process, brands can save time and resources that would otherwise be spent manually analyzing data and identifying influencers. This allows them to focus on other aspects of their marketing strategy and allocate their resources more effectively.
Automation also reduces the risk of human error and bias in influencer selection. Machine learning algorithms analyze data objectively and make decisions based on patterns and trends, reducing the risk of subjective judgments or biases.
Future of Influencer Marketing with Machine Learning
The future of influencer marketing with machine learning holds great potential for advancements and innovations. As technology continues to evolve, machine learning algorithms will become more sophisticated and capable of analyzing larger datasets more efficiently.
One potential advancement is the use of natural language processing (NLP) algorithms to analyze the content of influencers’ posts and comments. By understanding the sentiment and context of the content, machine learning algorithms can provide brands with valuable insights into how their target audience perceives their brand or products.
Another potential advancement is the integration of machine learning algorithms with other marketing technologies, such as customer relationship management (CRM) systems or marketing automation platforms. By combining data from different sources, brands can create a more holistic view of their customers and develop more targeted and personalized influencer marketing campaigns.
In conclusion, machine learning plays a crucial role in influencer marketing by helping brands identify and select the most suitable influencers, optimize campaign performance, and personalize campaigns. By analyzing large amounts of data quickly and accurately, machine learning algorithms can provide valuable insights and predictions that can help brands make informed decisions and achieve better results. As technology continues to advance, the future of influencer marketing with machine learning holds great potential for advancements and innovations that will further enhance the effectiveness and efficiency of influencer marketing strategies.
FAQs
What is machine learning-powered influencer marketing?
Machine learning-powered influencer marketing is a marketing strategy that uses machine learning algorithms to identify and target the most relevant influencers for a brand’s campaign. It involves analyzing large amounts of data to identify patterns and insights that can be used to optimize influencer selection, content creation, and campaign performance.
How does machine learning-powered influencer marketing work?
Machine learning-powered influencer marketing works by analyzing data from various sources, such as social media platforms, to identify influencers who are most likely to resonate with a brand’s target audience. This data is then used to create personalized campaigns that are tailored to the interests and preferences of the target audience.
What are the benefits of machine learning-powered influencer marketing?
The benefits of machine learning-powered influencer marketing include improved targeting and engagement, increased ROI, and better campaign performance. By using machine learning algorithms to analyze data and identify the most relevant influencers, brands can create more effective campaigns that resonate with their target audience and drive better results.
What are some examples of machine learning-powered influencer marketing?
Some examples of machine learning-powered influencer marketing include using machine learning algorithms to analyze social media data and identify the most relevant influencers for a brand’s campaign, using machine learning to optimize content creation and delivery, and using machine learning to track and measure campaign performance in real-time.
What are the challenges of machine learning-powered influencer marketing?
The challenges of machine learning-powered influencer marketing include the need for large amounts of data to train machine learning algorithms, the complexity of analyzing and interpreting data, and the potential for bias in the data and algorithms used. Additionally, machine learning-powered influencer marketing requires significant investment in technology and expertise, which may be a barrier for some brands.
Thanks for sharing. I read many of your blog posts, cool, your blog is very good.