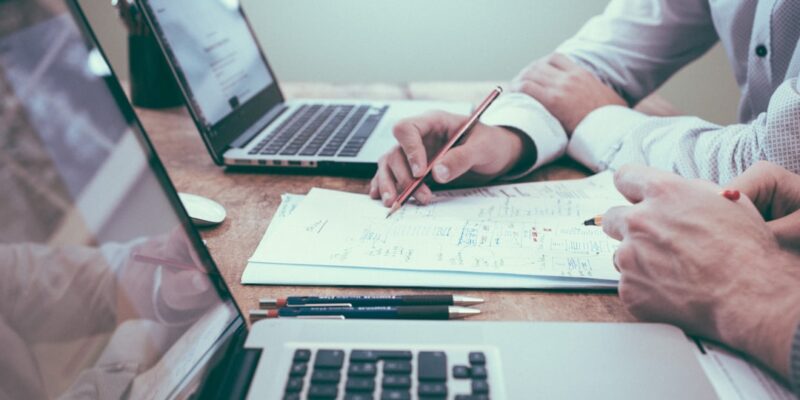
Revolutionize Your Pricing Strategy with Algorithmic Optimization
Algorithmic optimization refers to the use of mathematical algorithms and data analysis techniques to improve pricing strategies in business. Pricing strategies play a crucial role in determining the success of a business, as they directly impact revenue, profitability, and customer satisfaction. By leveraging algorithmic optimization, businesses can make more informed pricing decisions that are based on data-driven insights, leading to improved outcomes.
Algorithmic optimization can improve pricing strategies in several ways. Firstly, it allows businesses to optimize prices based on various factors such as demand, competition, and customer behavior. This helps in maximizing revenue and profitability by setting the right prices for products or services. Secondly, algorithmic optimization enables businesses to better understand their customers’ preferences and behavior, leading to improved customer satisfaction. Lastly, algorithmic optimization can help businesses gain a competitive advantage by positioning themselves effectively in the market.
Key Takeaways
- Algorithmic optimization can revolutionize pricing strategies
- Benefits of algorithmic optimization include increased revenue and improved customer satisfaction
- Data plays a crucial role in algorithmic optimization for pricing
- Implementing algorithmic optimization requires a combination of technology and human expertise
- Best practices for algorithmic optimization include continuous monitoring and testing
- Key metrics for measuring success include revenue growth and customer retention
- Case studies show that algorithmic optimization can lead to significant improvements in pricing strategies
- Common challenges include data quality and resistance to change
- Future trends include increased use of machine learning and AI in pricing optimization
- Algorithmic optimization is the future of pricing strategy.
Benefits of Algorithmic Optimization for Pricing
1. Increased revenue and profitability: Algorithmic optimization allows businesses to set prices that are optimized for maximum revenue and profitability. By analyzing data on demand, competition, and customer behavior, businesses can identify the optimal price points that will maximize their revenue. This can lead to significant increases in profitability and overall financial performance.
2. Improved customer satisfaction: Algorithmic optimization enables businesses to better understand their customers’ preferences and behavior. By analyzing data on customer demographics, purchase history, and browsing patterns, businesses can tailor their pricing strategies to meet the specific needs and preferences of their customers. This leads to improved customer satisfaction and loyalty.
3. Better market positioning: Algorithmic optimization helps businesses position themselves effectively in the market by setting prices that are competitive and attractive to customers. By analyzing data on competitor pricing, market trends, and customer behavior, businesses can identify the optimal price points that will give them a competitive edge. This allows them to differentiate themselves from competitors and attract more customers.
4. Competitive advantage: Algorithmic optimization gives businesses a competitive advantage by enabling them to make more informed pricing decisions. By leveraging data and analytics, businesses can gain insights into market trends, customer behavior, and competitor pricing strategies. This allows them to stay ahead of the competition and make pricing decisions that are based on data-driven insights.
Understanding the Role of Data in Algorithmic Optimization
Data plays a crucial role in algorithmic optimization for pricing strategies. There are several types of data that are used in algorithmic optimization, including historical sales data, customer data, competitor data, and market data.
Historical sales data provides insights into past pricing strategies and their impact on revenue and profitability. By analyzing this data, businesses can identify patterns and trends that can help inform future pricing decisions.
Customer data includes information on customer demographics, purchase history, and browsing patterns. This data helps businesses understand their customers’ preferences and behavior, allowing them to tailor their pricing strategies to meet their specific needs.
Competitor data provides insights into competitor pricing strategies and market trends. By analyzing this data, businesses can identify opportunities for differentiation and set prices that are competitive and attractive to customers.
Market data includes information on market trends, economic conditions, and industry benchmarks. This data helps businesses understand the broader market context in which they operate, allowing them to make more informed pricing decisions.
Data quality and accuracy are crucial for algorithmic optimization. Inaccurate or incomplete data can lead to incorrect insights and suboptimal pricing decisions. Therefore, it is important for businesses to ensure that their data is accurate, up-to-date, and reliable.
Data analysis techniques such as regression analysis, clustering analysis, and machine learning algorithms are used in algorithmic optimization to analyze and interpret the data. These techniques help businesses uncover patterns, relationships, and trends in the data that can inform pricing decisions.
How to Implement Algorithmic Optimization in Your Pricing Strategy
Metrics | Description |
---|---|
Conversion Rate | The percentage of website visitors who complete a desired action, such as making a purchase or filling out a form. |
Revenue per Visitor | The average amount of revenue generated by each website visitor. |
Customer Lifetime Value | The total amount of revenue a customer is expected to generate over the course of their relationship with a company. |
Cost per Acquisition | The amount of money spent on marketing and advertising to acquire a new customer. |
Profit Margin | The percentage of revenue that is profit after all expenses have been paid. |
Implementing algorithmic optimization in your pricing strategy involves several steps:
1. Before implementing algorithmic optimization, it is important to gather and analyze relevant data. This includes historical sales data, customer data, competitor data, and market data. By analyzing this data, businesses can gain insights into past pricing strategies, customer behavior, competitor pricing strategies, and market trends.
2. Choosing the right algorithm is crucial for successful algorithmic optimization. There are several algorithms that can be used for pricing optimization, including linear regression, decision trees, and neural networks. The choice of algorithm depends on the specific requirements and objectives of the business.
3. Integrating algorithmic optimization into existing pricing strategies requires careful planning and implementation. Businesses need to ensure that the algorithmic optimization process is aligned with their overall pricing strategy and business objectives. This may involve making changes to pricing processes, systems, and workflows.
Best Practices for Algorithmic Optimization in Pricing
To ensure the success of algorithmic optimization in pricing strategies, businesses should follow these best practices:
1. Regularly updating algorithms: Markets and customer preferences are constantly changing, so it is important to regularly update algorithms to reflect these changes. This involves analyzing new data, retraining algorithms, and fine-tuning pricing strategies based on the latest insights.
2. Testing and validating algorithms: Before implementing algorithmic optimization in a live environment, it is important to test and validate algorithms to ensure their accuracy and effectiveness. This can be done through A/B testing or by using historical data to simulate different scenarios.
3. Monitoring and adjusting pricing strategies: Once algorithmic optimization is implemented, it is important to continuously monitor and adjust pricing strategies based on real-time data and feedback. This allows businesses to quickly respond to changes in market conditions or customer behavior.
Key Metrics to Measure the Success of Algorithmic Optimization
To measure the success of algorithmic optimization in pricing strategies, businesses should track the following key metrics:
1. Revenue and profitability: Algorithmic optimization should lead to increased revenue and profitability. By comparing the financial performance before and after implementing algorithmic optimization, businesses can assess the impact on their bottom line.
2. Customer satisfaction and loyalty: Algorithmic optimization should lead to improved customer satisfaction and loyalty. By tracking metrics such as customer retention rate, customer satisfaction scores, and Net Promoter Score (NPS), businesses can assess the impact on customer relationships.
3. Market share and positioning: Algorithmic optimization should help businesses gain a competitive advantage and improve their market positioning. By tracking metrics such as market share, customer acquisition rate, and brand perception, businesses can assess their performance relative to competitors.
Case Studies: Companies that have Successfully Revolutionized their Pricing Strategies with Algorithmic Optimization
Several companies have successfully revolutionized their pricing strategies with algorithmic optimization. Here are three case studies:
1. Amazon: Amazon uses algorithmic optimization to dynamically adjust prices based on factors such as demand, competition, and customer behavior. By analyzing vast amounts of data in real-time, Amazon is able to set prices that are competitive and attractive to customers, leading to increased revenue and profitability.
2. Uber: Uber uses algorithmic optimization to dynamically adjust prices based on factors such as supply and demand, traffic conditions, and customer behavior. By analyzing data from its network of drivers and riders, Uber is able to set prices that are optimized for maximum revenue and customer satisfaction.
3. Netflix: Netflix uses algorithmic optimization to dynamically adjust prices based on factors such as content availability, subscriber behavior, and market trends. By analyzing data on subscriber preferences and viewing patterns, Netflix is able to set prices that are tailored to individual subscribers, leading to improved customer satisfaction and loyalty.
Common Challenges in Algorithmic Optimization and How to Overcome Them
There are several common challenges in algorithmic optimization for pricing strategies:
1. Data quality and accuracy issues: Inaccurate or incomplete data can lead to incorrect insights and suboptimal pricing decisions. To overcome this challenge, businesses should invest in data quality management processes and tools to ensure that their data is accurate, up-to-date, and reliable.
2. Lack of expertise in algorithmic optimization: Implementing algorithmic optimization requires specialized knowledge and skills in data analysis, statistics, and machine learning. To overcome this challenge, businesses can hire data scientists or work with external consultants who have expertise in algorithmic optimization.
3. Resistance to change: Implementing algorithmic optimization may require changes to existing pricing processes, systems, and workflows. This can sometimes be met with resistance from employees or stakeholders who are resistant to change. To overcome this challenge, businesses should communicate the benefits of algorithmic optimization and involve key stakeholders in the implementation process.
Future Trends in Algorithmic Optimization for Pricing Strategies
The future of algorithmic optimization for pricing strategies is likely to be shaped by the following trends:
1. Increased use of artificial intelligence and machine learning: Artificial intelligence and machine learning technologies are becoming more advanced and accessible, allowing businesses to leverage these technologies for algorithmic optimization. This will enable businesses to make more accurate predictions and recommendations based on complex data sets.
2. Integration with other business functions: Algorithmic optimization is likely to become more integrated with other business functions such as marketing, sales, and supply chain management. This will enable businesses to optimize pricing strategies in a more holistic and coordinated manner.
3. Personalization of pricing strategies: Algorithmic optimization will enable businesses to personalize pricing strategies based on individual customer preferences and behavior. This will allow businesses to offer customized pricing plans or discounts that are tailored to each customer’s needs.
Why Algorithmic Optimization is the Future of Pricing Strategy
In conclusion, algorithmic optimization is the future of pricing strategy due to its ability to improve revenue, profitability, customer satisfaction, and market positioning. By leveraging data and analytics, businesses can make more informed pricing decisions that are based on data-driven insights. This allows them to set prices that are optimized for maximum revenue and customer satisfaction, giving them a competitive advantage in the market. To stay ahead of the competition, businesses should adopt algorithmic optimization in their pricing strategies and continuously monitor and adjust their pricing strategies based on real-time data and feedback.
FAQs
What is algorithmic pricing optimization?
Algorithmic pricing optimization is the use of mathematical algorithms to determine the optimal price for a product or service based on various factors such as demand, competition, and market trends.
How does algorithmic pricing optimization work?
Algorithmic pricing optimization works by analyzing large amounts of data to identify patterns and trends that can be used to determine the optimal price for a product or service. This data can include information on customer behavior, competitor pricing, and market trends.
What are the benefits of algorithmic pricing optimization?
The benefits of algorithmic pricing optimization include increased revenue, improved profitability, and better customer satisfaction. By optimizing prices based on data-driven insights, businesses can ensure that they are charging the right price for their products or services, which can lead to increased sales and profits.
What are the challenges of algorithmic pricing optimization?
The challenges of algorithmic pricing optimization include the need for accurate data, the complexity of the algorithms used, and the potential for unintended consequences. Additionally, there may be ethical concerns around using algorithms to set prices, particularly if they result in price discrimination or other unfair practices.
What industries use algorithmic pricing optimization?
Algorithmic pricing optimization is used in a variety of industries, including retail, e-commerce, travel, and hospitality. It is particularly common in industries where prices are highly variable and subject to frequent changes based on market conditions and customer demand.